December 25, 2024|6 min reading
Fine-Tuning LLaMA Models with LLaMA-Factory: A Complete Guide
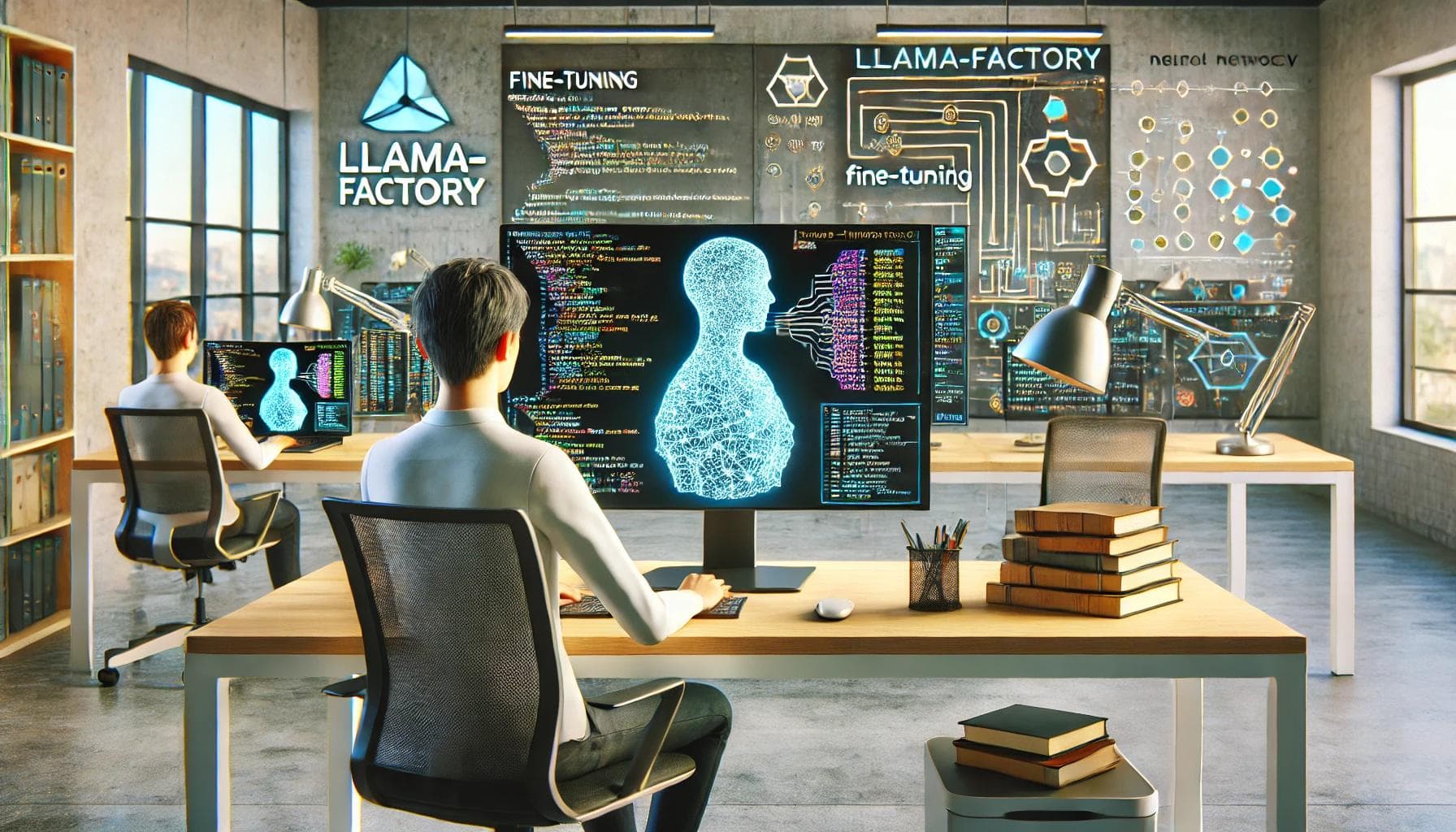
LLaMA-Factory is an open-source toolkit designed for fine-tuning, serving, and benchmarking LLaMA models, developed by Meta AI. With this step-by-step guide, you can unleash the full power of LLaMA models, fine-tune them for your use case, and deploy them effectively.
Introduction to LLaMA-Factory
LLaMA-Factory simplifies working with LLaMA (Large Language Model Adaptation), a family of large-scale language models developed by Meta. This open-source toolkit includes essential scripts for training, data processing, and benchmarking, enabling developers to quickly customize LLaMA models for their specific needs.
Key features of LLaMA-Factory:
- Data preprocessing and tokenization scripts
- Training pipelines for fine-tuning
- Inference scripts for text generation
- Benchmarking tools for performance evaluation
- Gradio web UI for real-time testing
In this guide, we’ll walk you through the process of setting up, fine-tuning, and deploying LLaMA models using LLaMA-Factory.
Setting Up LLaMA-Factory
Step 1: Create a Virtual Environment
Before starting, it's recommended to use a virtual environment to isolate dependencies. Here’s how to set it up:
bashCopy codepython -m venv llama-env
source llama-env/bin/activate # On Windows, use llama-env\Scripts\activate
Step 2: Install Dependencies
Install the required Python packages by running:
bashCopy codepip install -r requirements.txt
Ensure that you have access to LLaMA’s pretrained model weights, which are available through Meta’s research program. Once you have access, place the weights in the llama_checkpoints directory.
Preparing Data for LLaMA-Factory
LLaMA-Factory expects the training data in a specific JSON format. Each entry in the JSON file should include an instruction, an optional input, and the output (target response).
Example format:
jsonCopy code[
{
"instruction": "What is the capital of France?",
"input": "",
"output": "Paris is the capital of France."
}
]
To process and tokenize the dataset, use the following command:
bashCopy codepython data_preprocess.py --data_path data/alpaca_data.json --save_path data/alpaca_data_tokenized.json
This will tokenize your data using LLaMA’s tokenizer and save it in the required format.
Fine-Tuning LLaMA Models
Now that your data is prepared, you can fine-tune your LLaMA model using the finetune.py script. Here’s how to start fine-tuning:
bashCopy codepython finetune.py \
--model_name llama-7b \
--data_path data/alpaca_data_tokenized.json \
--output_dir output/llama-7b-alpaca \
--num_train_epochs 3 \
--batch_size 128 \
--learning_rate 2e-5 \
--fp16
Key parameters to adjust:
- model_name: The specific LLaMA model (e.g., llama-7b) you want to fine-tune.
- data_path: Path to the tokenized data.
- output_dir: Directory where the fine-tuned model will be saved.
- num_train_epochs: Number of training epochs.
- batch_size: The batch size for training.
- learning_rate: The learning rate for the optimizer.
- fp16: Enable FP16 for reduced memory usage.
Once training is complete, your fine-tuned model will be saved to the specified directory.
Performing Inference
With your fine-tuned model ready, you can generate text completions using the generate.py script:
bashCopy codepython generate.py \
--model_path output/llama-7b-alpaca \
--prompt "What is the capital of France?"
This command will load the fine-tuned model and generate a response based on the provided prompt.
Using the Web UI
LLaMA-Factory also offers a Gradio-based web UI, allowing for interactive testing. To launch the UI, use the following command:
bashCopy codepython web_ui.py --model_path output/llama-7b-alpaca
This will start a local server and open the UI in your browser. You can enter prompts and view real-time model responses.
Benchmarking LLaMA Models
LLaMA-Factory includes benchmarking tools to evaluate the performance of your fine-tuned models. Use the benchmark.py script to evaluate your model on different datasets:
bashCopy codepython benchmark.py \
--model_path output/llama-7b-alpaca \
--benchmark_datasets alpaca,hellaswag
This command evaluates the model’s performance on benchmark datasets like Alpaca and Hellaswag and outputs metrics such as accuracy and perplexity.
Conclusion
LLaMA-Factory provides a powerful and flexible toolkit for fine-tuning, deploying, and evaluating LLaMA models. With its easy-to-follow setup, comprehensive training pipelines, and benchmarking tools, you can quickly adapt LLaMA models for your own needs. Whether you're a researcher or a developer, LLaMA-Factory simplifies the process of creating cutting-edge AI applications.
To get started and explore more, visit the LLaMA-Factory GitHub repository:
GitHub - LLaMA-Factory
FAQs
What is LLaMA-Factory?
LLaMA-Factory is an open-source project that provides tools for fine-tuning, serving, and benchmarking LLaMA models developed by Meta AI.
How can I access LLaMA model weights?
The LLaMA model weights can be requested from Meta for research purposes. Once approved, you can place them in the llama_checkpoints directory.
What datasets can I use for fine-tuning?
You can use custom datasets or example datasets like Alpaca, which is included in the LLaMA-Factory repository.
Can I deploy my fine-tuned model?
Yes, you can deploy your model locally or on cloud platforms. LLaMA-Factory provides both Python scripts and a Gradio-based web UI for easy deployment.
4o
Explore more
How to Run Google Gemma Locally and in the Cloud
Learn how to deploy Google Gemma AI locally and in the cloud. A step-by-step guide for beginners and experts on maximizi...
How to Remove the Grey Background in ChatGPT: Step-by-Step Guide
Learn how to remove ChatGPT’s grey background with our step-by-step guide. Enhance your user experience with customizati...
Create AI Singing and Talking Avatars with EMO
Discover how EMO (Emote Portrait Alive) revolutionizes AI avatar creation, enabling singing and talking heads from a sin...