December 25, 2024|9 min reading
How to Fine-Tune LLaMA 3 Models: A Complete Guide
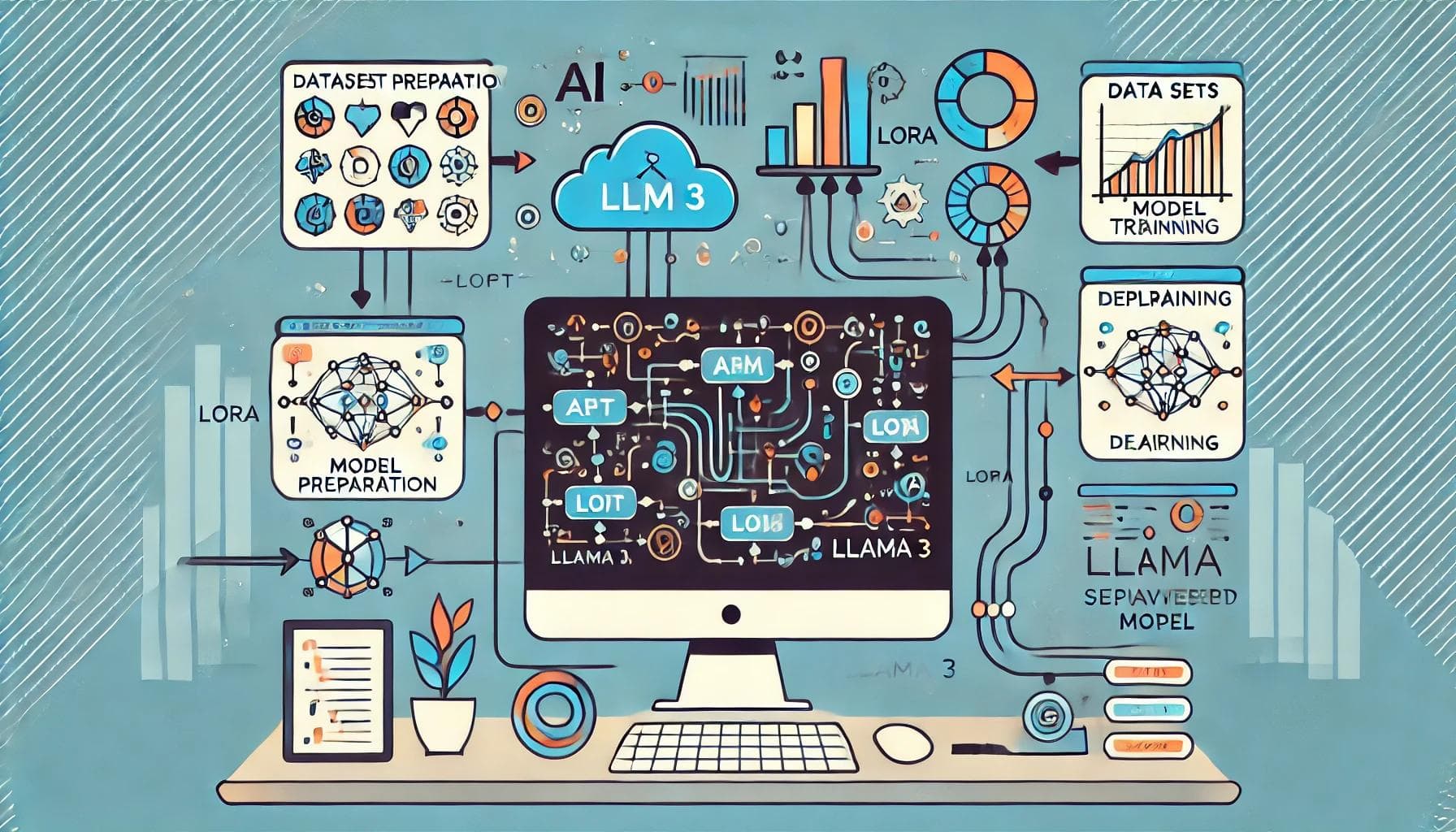
Don't Miss This Free AI!
Unlock hidden features and discover how to revolutionize your experience with AI.
Only for those who want to stay ahead.
Fine-tuning large language models (LLMs) like LLaMA 3 is essential to adapt them to specific tasks or domains. LLaMA 3, developed by Meta, is a state-of-the-art open-source model that can be customized to achieve impressive task-specific results. In this guide, we will walk you through the process of fine-tuning LLaMA 3, covering multiple methods, best practices, and resources to help you unlock the full potential of this powerful AI model.
What is LLM Fine-Tuning?
Fine-tuning is the process of taking a pre-trained language model and adapting it to perform well on specific tasks or datasets. This involves further training on a task-specific dataset to improve performance for the target application. Fine-tuning allows developers to leverage the general capabilities of large models like LLaMA 3 while specializing them for niche needs such as customer service, medical diagnostics, or legal document analysis.
Why Fine-Tune LLaMA 3? What's the Magic?
While LLaMA 3 is a powerful model out of the box, fine-tuning it can significantly enhance its accuracy and adaptability for specific use cases. Here’s why fine-tuning is worth the effort:
1. Improved Task Performance
Fine-tuning helps LLaMA 3 specialize in tasks like question answering, text summarization, or code generation, which leads to better performance and higher accuracy.
2. Domain Adaptation
By training the model on domain-specific datasets, such as medical or legal texts, LLaMA 3 becomes better at understanding and generating contextually appropriate content for that domain.
3. Customization
Fine-tuning allows for customization based on specific needs, such as incorporating stylistic preferences, tone, or specialized knowledge into the model’s output.
4. Resource Efficiency
Compared to training a model from scratch, fine-tuning uses fewer computational resources and is generally faster, making it a more accessible approach for many developers.
Methods for Fine-Tuning LLaMA 3
There are several methods you can use to fine-tune LLaMA 3. Depending on your coding skills and resource requirements, some methods may be more suitable than others.
Method 1: Fine-Tune LLaMA 3 with MonsterAPI (No Code Needed)
If you're not familiar with coding, MonsterAPI offers a simple, no-code solution for fine-tuning LLaMA 3. Here’s how to get started:
Create an Account: Sign up at MonsterAPI’s website.
Load the Model: Choose the LLaMA 3 model and load it with your task description.
Fine-Tune: Provide MonsterAPI with details about your task, and it will suggest a dataset and automatically fine-tune the model for you.
Deploy: After fine-tuning, you can deploy the model with a single click.
This method is perfect for non-developers or anyone who wants a fast, code-free solution to fine-tuning LLaMA 3.
Method 2: Fine-Tune LLaMA 3 with PEFT and Hugging Face
For developers looking for more control over the fine-tuning process, using PEFT (Parameter-Efficient Fine-Tuning) along with the Hugging Face library is an excellent choice. PEFT methods, such as LoRA (Low-Rank Adaptation), allow you to update only a small portion of the model’s parameters while keeping the majority of the model frozen, saving both time and resources.
Here’s how to fine-tune LLaMA 3 using PEFT and Hugging Face:
pythonCopy codefrom transformers import AutoTokenizer, AutoModelForCausalLM, TrainingArguments, Trainer
from peft import LoraConfig, get_peft_model
# Load pre-trained LLaMA 3 model and tokenizer
model_name = "decapoda-research/llama-3-8b-hf"
tokenizer = AutoTokenizer.from_pretrained(model_name)
model = AutoModelForCausalLM.from_pretrained(model_name)
# Define LoRA configuration
lora_config = LoraConfig(
r=16,
lora_alpha=32,
target_modules=["q_proj", "v_proj"],
lora_dropout=0.05,
bias="none",
task_type="CAUSAL_LM"
)
# Apply LoRA to the model
model = get_peft_model(model, lora_config)
# Prepare the dataset
dataset = ...
# Define training arguments
training_args = TrainingArguments(
output_dir="./lora_llama3",
per_device_train_batch_size=4,
num_train_epochs=3,
learning_rate=1e-4,
)
# Fine-tune the model
trainer = Trainer(
model=model,
args=training_args,
train_dataset=dataset,
)
trainer.train()
This approach provides fine-grained control and flexibility over your fine-tuning process, making it suitable for advanced users.
Method 3: Fine-Tune LLaMA 3 with Unsloth Library
Unsloth is an efficient library that simplifies the fine-tuning process for LLaMA 3. It optimizes memory usage and accelerates training times, making it a fantastic choice for large-scale models. Here's how to set up your environment and fine-tune LLaMA 3 with Unsloth:
Setting Up the Environment
pythonCopy codeimport torch
from unsloth import FastLanguageModel
# Set parameters for fine-tuning
model, tokenizer = FastLanguageModel.from_pretrained(
model_name="unsloth/llama-3-8b-bnb-4bit",
max_seq_length=2048,
dtype=None,
load_in_4bit=True,
)
Adding LoRA Adapters
pythonCopy codemodel = FastLanguageModel.get_peft_model(
model,
r=32,
target_modules=["q_proj", "k_proj", "v_proj", "o_proj"],
lora_alpha=32,
lora_dropout=0.1,
use_gradient_checkpointing="unsloth",
)
Training the Model
pythonCopy codefrom trl import SFTTrainer
from transformers import TrainingArguments
trainer = SFTTrainer(
model=model,
tokenizer=tokenizer,
train_dataset=dataset,
dataset_text_field="text",
args=TrainingArguments(
per_device_train_batch_size=4,
num_train_epochs=3,
learning_rate=1e-4,
),
)
trainer.train()
Unsloth provides memory-efficient and fast fine-tuning, especially when dealing with large models like LLaMA 3.
Best Practices for Fine-Tuning LLaMA 3
Regardless of the method you choose, following best practices can make a significant difference in the outcome of your fine-tuning process:
Data Quality: Ensure that your dataset is clean, well-labeled, and representative of the task you are fine-tuning the model for.
Hyperparameter Tuning: Experiment with different learning rates, batch sizes, and other training parameters to find the optimal setup.
Evaluate Regularly: Periodically evaluate the model’s performance on a validation set to prevent overfitting and ensure generalization.
Resource Management: Fine-tuning large models can be resource-intensive. Use techniques like mixed precision training and gradient checkpointing to save memory and speed up training.
Conclusion
Fine-tuning LLaMA 3 can significantly enhance its capabilities for specific tasks, making it more efficient and accurate. Whether you're a beginner looking for a code-free solution with MonsterAPI, or an advanced user interested in fine-tuning with PEFT and Hugging Face, there’s a method for everyone. By following the best practices outlined above, you can maximize the impact of your fine-tuned model.
SEO FAQ
Q1: What is LLaMA 3?
LLaMA 3 is a large language model developed by Meta. It is open-source and capable of performing a wide range of tasks like text generation, summarization, and translation.
Q2: Why should I fine-tune LLaMA 3?
Fine-tuning allows you to adapt LLaMA 3 to specific tasks or domains, improving its performance and making it more efficient for your needs.
Q3: What is PEFT?
PEFT (Parameter-Efficient Fine-Tuning) is a technique that reduces the number of parameters being trained, making the fine-tuning process more efficient and less resource-intensive.
Q4: How can I deploy my fine-tuned LLaMA 3 model?
After fine-tuning, you can save and deploy your model for real-time applications using APIs or integrate it into your workflow with platforms like Hugging Face or MonsterAPI.
OChatGPT can make mistakes. Ch
Explore more
Top 12 HeyGen AI Alternatives for 2025
Explore the 12 best HeyGen AI alternatives in 2025 for AI video generation. Find powerful platforms for realistic avatar...
Top 10 Free AI Voice Generators for Lifelike Audio
Easily convert text to speech, create realistic voiceovers for videos, podcasts, and more with these top tools
Claude MCP Server: Revolutionizing AI Interaction & Data Access
Discover how Claude MCP Server standardizes AI interaction with external data & tools. Learn its benefits for enterprise...